Large Language Models (LLM), such as GPT-4, have taken over the tech sector at a breakneck speed. While this technology has enormous potential, it also creates a new attack surface that security organizations will have to protect. This new attack surface is already exposed in most organizations and therefore, this blog will help mitigate these incidents through:
· Identifying risk in the following categories: trust & safety, data privacy, third-party risk, and supply chain risk.
· Proposing risk mitigation strategies that security teams can employ.
1. Trust and Safety Risk
Numerous stories have been written about positive and negative outputs of text-based language models. Accelerated software development, summarization of long-form text, and question-answering are hailed as breakthroughs that will enhance human productivity. Unfortunately, fake citations, buggy code, and misstated facts are also common.
RISK: Embedding or providing access to LLM capabilities can produce responses misaligned with an organization’s values. Common examples of this include:
· Instructions on how to harm one self or others
· Information that may enable an individual to bypass organizations’ security controls
· Hate speech targeting specific groups or individuals
IMPACT: Potential legal liability and damaging brand reputation.
MITIGATION: Prompt inspection: An organization can invest in tools either native to the AI platform or placed in line with generative AI tools that will inspect user prompts and block input types that may lead to undesired outputs. Several vendors have emerged to provide this capability, including:
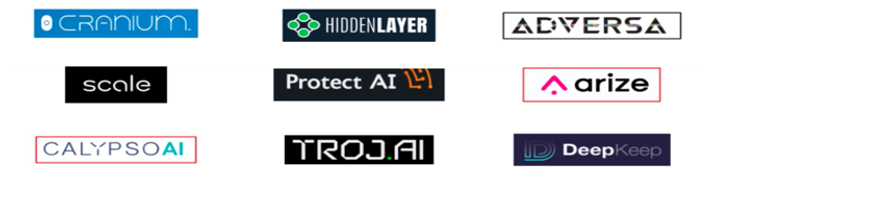
2. Data Privacy
LLM models rely on extensive datasets as input for their training process. Models are continuously fine-tuned through reinforcement learning where new data samples are added to further influence how a model responds to specific prompts.
RISK: Company data uploaded to LLM services could become a part of the training data set, which could cause the LLM to expose sensitive company information to external parties.
IMPACT: A major tech company reported private company information was compromised when ChatGPT reproduced company data when prompted.
MITIGATION: Remote Browser Isolation technology can allow an organization to limit access to generative AI services by limiting activities such as file uploads and copy and paste to popular AI services. Many RBI vendors exist, including Island, Ericom, Red Access, and Skyhigh.
3. Third-Party Risk
Practically every technology company is moving fast to answer questions around AI strategy and risk mitigation techniques. One of these such questions revolves around the fact that existing data processing agreements may not have included language around consumer data processing by third-party AI providers.
RISK: Terms of service of many existing service providers are being updated to allow those products the right to incorporate AI capabilities via third-party data processors.
IMPACT: During a live presentation of this material, a case study was shared where an organization only became aware of new AI capability when on an unrelated service call with a vendor. This activity is not covered by their existing services agreement.
MITIGATION: Assume your existing technology services are adding AI capabilities. It’s prudent to closely monitor the changing terms of service for providers that process sensitive or protected company data.
4. Supply Chain Risk
It’s estimated that over 80% of all generative AI solutions will leverage pre-trained models. The data used to train these models is now a component of an organization’s supply chain because manipulation of the data used to train these models will impact the model’s behavior for end users.
RISK: An attacker inserts malicious data into the training process of a model provided via an AI service or published on a service such as HuggingFace. This causes a codeless vulnerability in an application that uses this pre-trained AI, which the attacker can later exploit.
IMPACT: An analog to intentional model manipulation is unintended drift. This is where an AI model substantially declines in its ability to perform a task. The figure below shows the changeover time of two models’ ability to answer a question.

MITIGATION: This is the most challenging risk to mitigate because you typically won’t have any visibility into the training data or reinforcement learning process. The best practice may be to leverage only models from trusted vendors and limit user access to open-source AI models on services such as HuggingFace.
This blog is derived from a talk given at a recent ISSA event, titled: A cyber leaders guide to LLM security.